Galdi's Research Recognised by the Scientific Community
A study on how to improve the efficiency of Galdi's diagnostic systems has been published in one of the prestigious scientific journals of the Institute of Electrical and Electronics Engineers (IEEE). Combining advanced diagnostics, Neural Networks and Machine Learning!
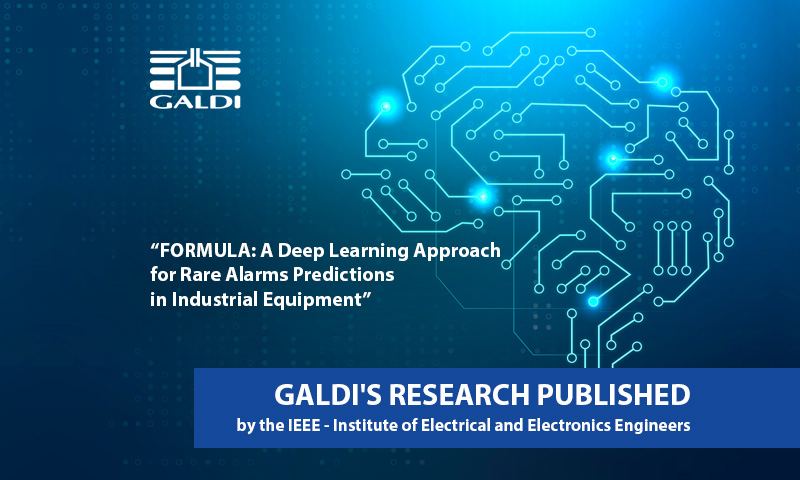
Can the issues having the most impact on filling machines be predicted?
In order to answer this question, a predictive model was created, which works for Galdi fillers but can be applied to several other sectors too.
The article "FORMULA: A Deep Learning Approach for Rare Alarms Predictions in Industrial Equipment" was published in the IEEE Transactions on Automation Science and Engineering journal, one of the world's leading sources for technical and scientific research. It was written jointly by:
- Diego Tosato, Galdi S.r.l.
- Davide Dalle Pezze, University of Padua
- Chiara Masiero, Statwolf Data Science S.r.l.
- Gian Antonio, Susto University of Padua
- Alessandro Beghi, University of Padua
GALDI'S RESEARCH WORK: FOCUS ON ADVANCED DIAGNOSTICS AND IIOT
For some years now, Galdi has been working on digitalisation and data analysis to enhance diagnostics on food filling machines.
This ongoing Research and Development work goes hand in hand with the evolution of Industrial Internet of Things (IIOT) solutions.
Our next-generation packaging machines are now equipped with new sensor systems connected to the performance monitoring platform MaSH, a system designed to predict potential anomalies to avoid unexpected downtime.
MACHINE LEARNING AND NEURAL NETWORKS FOR FILLING MACHINES
Today, the quantity and quality of data collected and sent by Galdi fillers enable us to have a detailed overview of the machine's actual performance, including accurate MME and OEE metrics.
On a deeper level, that same data allows Galdi engineers to have a global and detailed picture, which is useful for troubleshooting purposes, but also to predict potential anomalies.
And yet, from a predictive maintenance perspective, how can we predict the most serious alarms - e.g. dispenser membrane damage posing a contamination risk - which are rare events and therefore fail to generate a recurrent pattern that can be detected by the system?
By comparing datasets from different filler models, the researchers involved in the study came up with a solution based on Deep Neural Networks, a sub-field of Machine Learning.
This neural network model "absorbs information and learns" from collected datasets, and predicts what will happen in the future, generating the sequence of future alarms on the machine.
UNIVERSALLY SHARED INNOVATION
The contents of the research attracted considerable interest amongst the scientific community, given the little literature available on the subject.
The model is generalizable to a number of industrial contexts and can be applied to any machine generating log errors.
The ALPI (Alarm Logs in Packaging Industry) dataset containing the results of the study has been anonymised and made available in open source form, as both researchers and Galdi share the same philosophy: "The only way to drive innovation is to share it!"
SUPPORTING GALDI CUSTOMERS THROUGH APPLIED RESEARCH
The quality of the data on machine performance is an added value for our customers and an additional tool to monitor production.
Since reading the data is time-consuming and correct interpretation requires a lot of experience, we are developing a proactive service dedicated to our customers.
This is going to be a Data Consulting service to translate data into valuable information, both to predict potential problems and improve performance by analysing the system's Key Performance Indicators (KPI).
This additional tool brings us even closer to our customers and enhances our digital assistance services offering.
For more information,